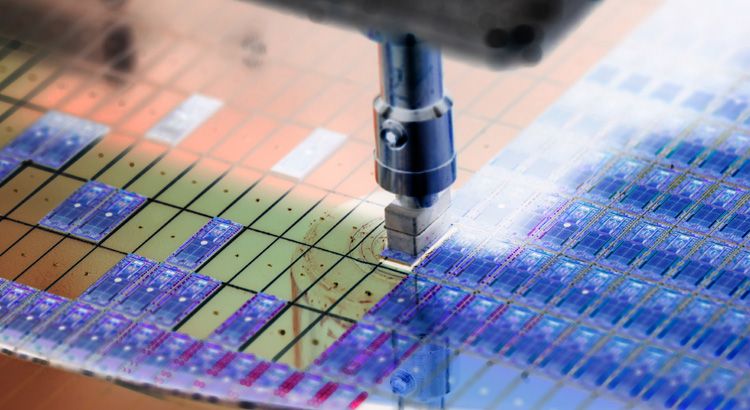
How Mercury Systems and Intel Collaborate to Bring Artificial Intelligence to Defense
Mercury Systems
September 3, 2020
Sensor modernization over the last decade has created an explosion of high-quality data and a need for AI algorithms to close the gap between receipt of data and the ability to act on it. Breakthroughs in software and hardware technologies are supporting the complex neural networks to run those AI algorithms. Hear from Mercury’s Devon Yablonski and Intel’s John Brynildson how Mercury and Intel collaborate, bringing their own unique sets of technological know-how, to develop and implement AI applications for quicker deployment and integration in the field to enhance national security.
Read the transcript.
Ralph Guevarez:
Hello, and welcome to Mercury Now, a podcast series brought to you by Mercury Systems. I am your host, Ralph Guevarez, and today’s topic. How Mercury and Intel collaborate to bring artificial intelligence to defense. Joining me is Devon Yablonski, embedded processing principal product manager here at Mercury Systems, and a special guest John Brynildson, who is the senior segment marketing manager in the IOTG sector at Intel. John, Devon, good day and welcome.
John Brynildson:
Thanks, Ralph.
Ralph Guevarez:
John, we appreciate you joining us today. Could you please give our listeners a brief background on your current role at Intel?
John Brynildson:
First, thank you very much for inviting me to join in on the podcast today. So I am with Intel corporation and I’ve been a part of Intel for a little over four years now. I’ve held a variety of roles, primarily in the aerospace and defense area, being Intel promote our efforts within this market’s vertical segment.
John Brynildson:
I have worked in sales roles and for the last year I’ve been part of the Internet of Things group as a senior marketing manager, helping again with the products that Intel creates for what we call our public sector market verticals.
Ralph Guevarez:
Thank you, John. Devon, a brief background.
Devon Yablonski:
Thanks, Ralph. I’m Devon Yablonski and I am a product manager in our embedded product group here at Mercury. I’ve been here since about 2011 and started in the engineering department and then worked through various functions of our product area, always focusing on our high performance signal processing and now artificial intelligence processing products.
Ralph Guevarez:
John, artificial intelligence, or AI implementation seems a top priority for the defense industry. What is driving this push and why is the defense industry adopting it?
John Brynildson:
Actually I think it’s a multi-faceted answer. I mean, there are multiple factors that are really driving the need for artificial intelligence. On one side there’s the sensor modernization’s gone on the past 10 years have really created an explosion of data, high quality data that is a good thing, but it also proposes many challenges for the defense industry, essentially really having enough resources to digest a lot of that data.
John Brynildson:
And so one of the many use cases that we’ve seen in the defense industry is using and developing AI algorithms or machine learning algorithms to really help close that gap between the receipt of data and to where you can make some kind of decision with that information.
John Brynildson:
So it’s impossible for many analysts in the defense industry to really comb through all the data that they get over in a day and using these techniques to help streamline that information and enable them to make a more effective decision has been one of the really compelling use cases that has been happening in defense industry.
John Brynildson:
And then the other element I really would like to touch on is the fact that there is really this enormous breakthrough in different technologies, both software and hardware technologies that are really allowing these complex neural networks to run these AI algorithms. So really that explosion of data, as well as the technologies that are really finally allowing this to result in some meaningful information for our partners in the defense industry, I think have been some couple key elements.
Ralph Guevarez:
Thank you, John. Now, Devon, everything is getting smarter with AI. We’re seeing evidence of that in our vehicles, smart devices, smart phones, and speakers. How does that translate to the defense space?
Devon Yablonski:
Well, as you’re seeing in aerospace and defense, they’re also investing in many of the same types of technologies using AI to make the warfighters more efficient and enhance national security. So we’re seeing this in every type of asset out there from air, to ground, to sea and a huge drive is that big data wave that John was talking about, which is coming from an explosion in the amount of sensors and the autonomy that we want to add. And that’s all about allowing for less human interaction, allowing for less skilled remote control operators among manned vehicle, and ultimately it’s all to limit the likelihood of human error and often to react more quickly to the emerging threats.
Devon Yablonski:
This demand’s really driven by the artificial intelligence advancements in the commercial and consumer industry over the past 5 to 10 years. Defense space without question kind of requires a higher quality result in most other areas in consumer world with the criticality of our missions and that’s why we’re just starting to see it play a more dominant role in our space.
Devon Yablonski:
The algorithms are finally reaching a point where they’re able to make really intelligent, reliable learnings and inference in near real time. And Intel’s product lines with their CPUs, FPGAs, artificial intelligence, ASICs like the Movidius chip and others, they’re really key to executing on that. And the translation really needs to happen fast. The other nations are rapidly adopting these capabilities and demonstrating their effectiveness.
Ralph Guevarez:
Now you mentioned developing and deploying technology at speed. My question is how are Intel and Mercury uniquely positioned to support AI applications? John, we’ll start with you.
John Brynildson:
Really Intel’s perspective is there is no one size fits all solution for every AI algorithm or every AI application and I think one of the unique offerings Intel brings is really that broad set of hardware solutions that allow customers to develop systems and platforms that meet their individual size, weight, and power, ruggedization features for their specific environment.
John Brynildson:
And as Devon mentioned it’s not just Intel CPUs, right? We have a whole portfolio of products, including FPGAs, our accelerators and then one of the other key elements is the software technologies that helps tie all that together. We believe Intel is very unique in a breadth of offering between hardware and software to really allow our customers to develop and implement their AI applications.
Ralph Guevarez:
Thank you. Devon, your input.
Devon Yablonski:
So the defense market has very similar capabilities as the consumer product world, but our challenges are significantly different. We have to account for a much harsher physical environments, radically different attack vectors and higher stakes on those results and meeting these has always traditionally meant a lot of development time to make a deployable system.
Devon Yablonski:
So at Mercury, where we’re uniquely built the capability to make commercial data center and cloud capabilities, like what is needed for artificial intelligence profoundly accessible to this market. And we do that within what I like to call the period of technological relevance. This is the time for when a technology like a new AI algorithm and chip is deployable out into an integrated solution in the field and considers both when it’s likely to be dated by the newer capabilities that are out there that would render it irrelevant and also when the adversaries would deploy similar capabilities or countermeasures.
Devon Yablonski:
But we need to be able to take this great processing storage, networking technologies and add all those features of ruggedization security safety certifications in time for it to be relevant. And one of the most critical aspects of this is getting early access to the latest and greatest Silicon to begin to our development of those capabilities.
Devon Yablonski:
So the partnership with Intel has really enabled us to bring their latest technology to benefit the warfighter faster than most others can. We can do a lot with even just early samples that aren’t even functional to help us develop mechanical and electrical solutions so that when technology becomes available, we can deploy it into our environments really quickly.
Ralph Guevarez:
Thank you. Now, Intel and Mercury are working together to bring the latest commercial data center processing to the fog and the edge. Can you speak more on that? John, we’ll start with you.
John Brynildson:
One of the things that, and Devon touched on this previously is that close interaction between our organizations. To rapidly integrate and deploy AI COTS technologies into the field, we collaborate very closely on our roadmaps, we collaborate on providing early to a lot of the products before they’re genuinely available. So that would enable Mercury to implement and develop their products in line with our product development.
John Brynildson:
And I think really more importantly is we have a very close engineering relationship. We seek quite closely development engagements around Intel’s hardware-based security. That depth of knowledge is critical for Mercury to understand how that works so they then can integrate that with your own unique IP expertise and ultimately giving the customer a more secure product.
John Brynildson:
One of the other areas that we engage very deeply on is also in the area of plate safety processing, where to have a Intel multi-core CPU that is for flight safety processing applications really requires a very in depth knowledge and understanding of Intel products. And that’s the level of relationship we have with Mercury to really give the end user in the defense industry that end product that will work in their multitude of different applications.
Ralph Guevarez:
Now, John, what security concerns does artificial intelligence raise?
John Brynildson:
Well, there’s quite a few actually. The two that really kind of stand out for me is the, what we call trusted AI. As a user is relying on AI algorithm how do they know that the results that they’re getting, that that AI algorithm may say, “Hey, that’s a truck.” How do they know that that is real, it hasn’t been tampered with?
John Brynildson:
One of the other safety concerns is types of products that Mercury develops are in that edge environment. They’re not in a secure data center with guards and fences around them. These are out in the field where they could easily be accessed by adversaries. And so with that in mind, how do you ensure that those adversaries can’t get into the hardware and back reverse engineer how that algorithm works? So either they steal that information for themselves or figure out how to counter that type of information. So, those are two big concerns that we hear about certainly and from the defense market segment.
Ralph Guevarez:
Thank you. Now, Devon, staying with the security concerns, there have been reports of widely accessible, unregulated commercial technologies being harnessed by unauthorized individuals and exploited through vulnerabilities. What must be done to alleviate those concerns?
Devon Yablonski:
Yeah, Ralph, these concerns are really important. And John started touch on it there. I find artificial intelligence really interesting around this because what you’re doing is you’re creating a solution that to varying degrees can think like a human that was trained on an extraordinarily amount of data, more than a human could actually ever process.
Devon Yablonski:
And most of that data in these cases will have probably been classified in nature or various strategic. Fortunately deep neural networks that are used for artificial intelligence today, they do obfuscate the data that’s put into them pretty well. The actual model, it’s hard to decipher what’s inside of it, but you can imagine if you could pick up that equipment and find out what the inputs and outputs were, you could realistically steal that brain and that contains all of that information balled inside of it. And that’s even more valuable than the data that was until now, pretty unmanageable. So you can see how that security threat’s even more real today.
Devon Yablonski:
In terms of protecting from that threat commercial consumer technologies are very different. Most of the protection in the cloud and data center are from cybersecurity hacks over the internet or physically loading of software onto the device. Most of the assets we work on are not connected to those wide area networks. And at the end of the day, they’re very likely to end up actually physically in an adversary’s session, so that’s the threat we’re trying to protect from.
Devon Yablonski:
And processing systems, therefore must be totally trusted. Trusted hardware, software, middleware, the integration and the support of it, and being able to do all that securely really mitigates the risk of those hardware vulnerabilities, impacting the product quality or reliability.
Ralph Guevarez:
Thank you, Devon. John, what bottlenecks must be overcome to make AI enabling edge hardware?
John Brynildson:
Certainly there are a couple key ones. I know that one of the key challenges is the end user may have a variety of different hardware solutions that they’re deploying at the edge having to prune and tuned or algorithms for each unique hardware application is a lot of work. And personally from the Intel perspective, we believe software is a key element in that and having a unified software stack that will allow you to take your models and optimize it and run across different hardware solutions is a key element.
John Brynildson:
Ultimately it comes back to how do we make it easier for the end user to deploy their AI algorithms at the edge? And there’s a lot of work going into that area. Certainly we believe the software is certainly a key element in making that better, easier to use is certainly one key element to have more end users deploy AI algorithms at the edge.
Ralph Guevarez:
Thank you, John. Devon, your thoughts.
Devon Yablonski:
Our market really requires more performance at the edge than most others you see. Networks to larger compute platforms and data centers are really not that reliable in the defense in the field and they’re a key target. So we need to assume that our endpoints need to have a fair amount of fully independent function.
Devon Yablonski:
The sensors are capturing data at higher resolution farther and wider, and in many spectrums compared to even what you see in some of the advanced self-driving vehicles in the commercial world today. So our customers are demanding for this data center capability, like what Intel and other Silicon vendor partners offer at size weight and power constraint endpoints, and an embedded, and embedded plus kind of environment.
Devon Yablonski:
So for example, the Intel Xeon scalable processors, those are the highest performance Xeons that Intel offers in the data center. And those typically aren’t thought of as an embedded processor. Our customers are asking for that because they can’t actually send data back to a data center with those processors. They need to potentially have that capability on the platform. And that’s the same for high-performance FPGAs and other types of accelerators.
Devon Yablonski:
So it’s imperative that the ruggedization and the other security and safety aspects we apply to these products don’t negatively impact their performance either, but still ensures that survivability in those austere field environment.
Ralph Guevarez:
Now, Devon, how do commercial off-the-shelf technologies COTS we mentioned earlier, simplify integration and improve interoperability?
Devon Yablonski:
So fundamentally COTS products and modular open standards are together about multiple vendors coming together to build a best of breed solution that is capable, cost effective, and can be maintainable and upgradable throughout what is typically a long, useful life cycle.
Devon Yablonski:
As a subsystem design and integrator like us at Mercury leveraging these standards allow us to build the right system for the need from a huge ecosystem of trusted hardware, software, and tools, both that we create as well as others. Within the product lines we offer, we focus on creating leverageable commercial off-the-shelf products from the scale of components, technology, building blocks, and then multi-use modules to design and integrate these subsystems to meet the latest commercial off-the-shelf standards.
Devon Yablonski:
And this milk methodology allows us to develop a high performance system that meets both today’s and tomorrow’s needs. And by using these commercial off-the-shelf solutions, we’re also able to leverage commercial investments and capabilities like what Intel provides, because they will be code and performance portable to our end solution.
Ralph Guevarez:
Thank you, Devon. Now, John, give our listeners a glimpse into the future. What are Intel and Mercury working towards to support the AI evolution?
John Brynildson:
As you look out where the AI evolution is going, one of the key characteristics certainly is performing a lot of your AI algorithms at the edge and certainly being at the edge there are a variety of end use applications and needs, the environments are very different. Devon talked about that.
John Brynildson:
One of the things we’re looking for in the future is having more products available that can meet a wider variety of end use field conditions. So, say for example, extended temperature, which would allow our partners like Mercury to have a more robust ruggedized end product with a variety of different elements. If those elements are the FPGAs or CPUs or any kind of accelerator.
John Brynildson:
That’s one thing that Intel is looking, is we look out in the future. I think the other that is pretty obvious is really building in more acceleration AI algorithm, acceleration capabilities into our products and some items that have been that are out already include Intel deep learning boost. There’s vector neural network instruction sets of VNNI and AVX, really things that can be used to help the acceleration of the algorithms.
John Brynildson:
Deep learning boost is a great example of a built in accelerator that can be leveraged within Intel products. So do you really need to have another component on your board when you have that already within your Intel product? So, that’s example of what’s there today, and I can tell you that there are more things to come in that area, so that is certainly one area that Intel is putting a lot of efforts into as we expand out our products over the next 5 to 10 years.
Ralph Guevarez:
Thank you, John. That’s very exciting. Devon, your thoughts.
Devon Yablonski:
Working with Intel has been amazing for the past decade or so of products we’ve been developing with them and we do expect that to just to get even better over time. I think we’re expecting to continue to integrate the highest performance processing memory networking and even storage capabilities in these secure, safe, and low size, weight, and power environments we’ve been discussing.
Devon Yablonski:
This will involve more dense capabilities than ever and really stretching the limits there, handling more challenging requirements like temperature john was talking about and more advanced shock and vibe, and really being able to, at the end of the day, turn the screws on being able to develop solutions more quickly. This artificial intelligence market, they say it’s doubling every three months and that means we really need to get our products out there quicker, so we’re going to find every way we can do that together and Intel’s a great partner in that regard.
Devon Yablonski:
Another interesting step, John did talk about OpenVINO earlier, and I think we’re seeing that there’s a need for more integration of the development and runtime tools that support artificial intelligence. Intel’s OpenVINO is particularly interesting to us because in order to provide efficient code solutions, so really to drive down that size, weight, and power of a system, that software needs to be efficient. And there’s a lot of different kinds of processor technology, FPGA accelerators that are all good for certain purposes, and we need to make the best use of those.
Devon Yablonski:
So if we can enable our customers to be able to develop and run their application with optimal efficiency, that’s going to be really powerful. So I’m looking forward to more integration of that layer of the software stack to support our customers.
Ralph Guevarez:
Gentlemen, I’d like to take this opportunity to thank you both for joining me today. I look forward to the progress with the Intel and Mercury relationship. Once again, thank you both for your time. I wish you the best of luck moving forward and Godspeed. Thanks again.
John Brynildson:
Thank you very much, Ralph.
Devon Yablonski:
Thank you, Ralph.
Ralph Guevarez:
This has been another edition of Mercury Now, the podcast series brought to you by Mercury Systems. I’m your host, Ralph Guevarez signing off.